Data is the raw material for machine learning projects. It must have the right:
Quantity: the more the data the better the performance of a machine learning model on the evaluation metric, i.e., accuracy, error rate, etc, and
Quality: the data must be clean, relevant to the problem and available when needed
While machine learning algorithms steal the limelight, data and its proper preparation drive much more value than algorithms. As a general rule, lots of good data run on a dumb algorithm produces better results than modest data run on a sophisticated algorithm, Figure 1 below.
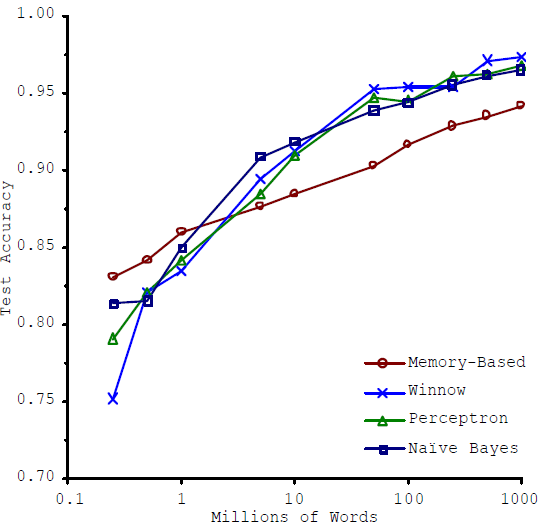
Source: “Learning Curves for Confusion Set Disambiguation”. Banko, M and Brill, E (2001), Scaling to Very Very Large Corpora for Natural Language Disambiguation